AI Assisted Consumer Listening - A Case Study
by Julia Valchanova
1. AI Assisted Processing
Most businesses intuitively understand the value of listening to their customers and making an effort to collect feedback. You've probably seen an email come through after using an online service that asks you to share your experience, what you liked and disliked. It's a great initiative, but the reality is that most businesses don't know how to draw value from this data. And that's a huge missed opportunity because this data is a gold mine. Having an unbiased view of what your customers think of your product and how it compares to your competitors is essential to navigating your business well.
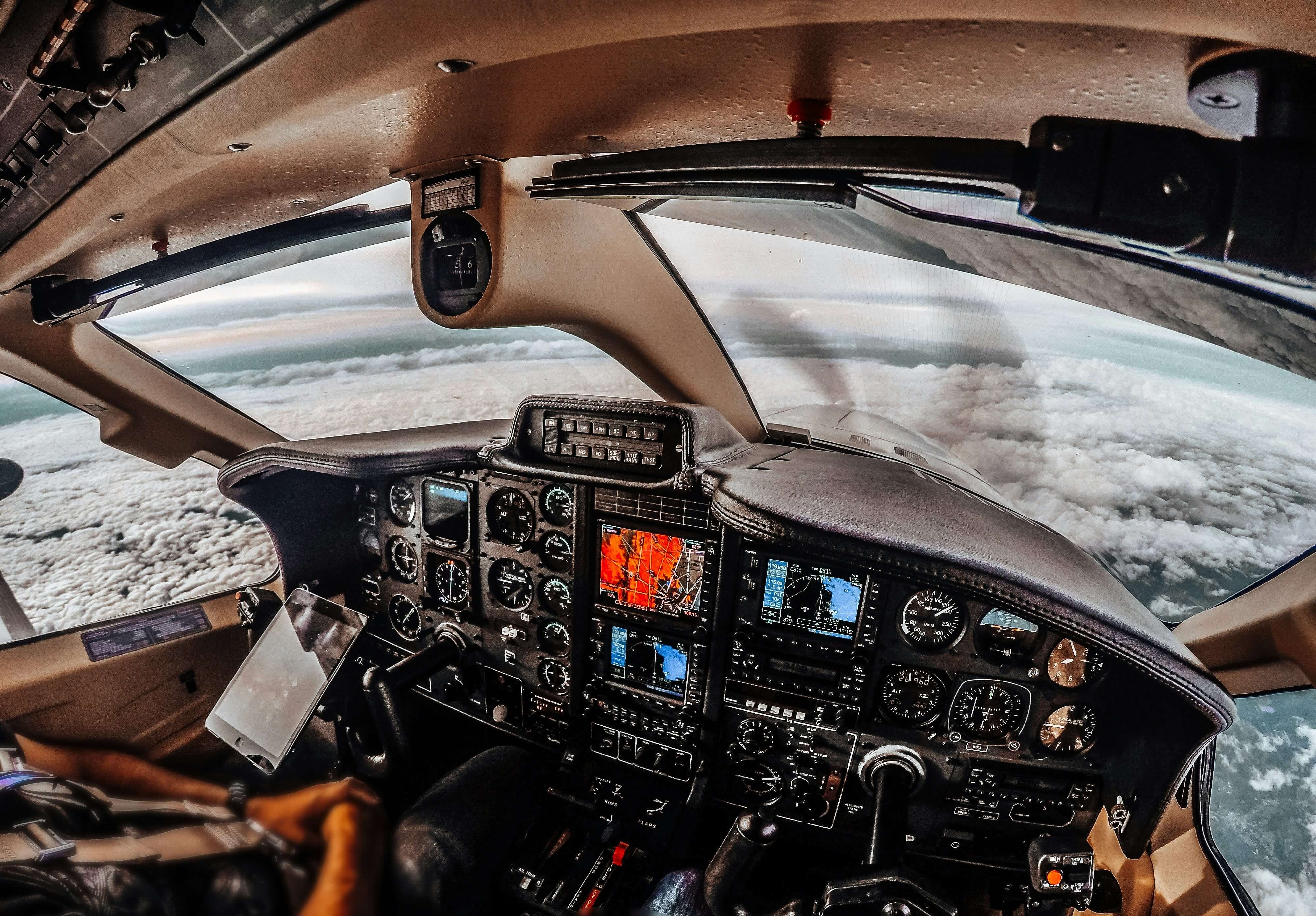
The kind of open-ended feedback that customers provide or leave as a comment spontaneously under a video or an article online is as authentic as feedback gets. However, interpreting this feedback in a systematic way that removes bias and works at scale when you have thousands of comments to work through is beyond human capabilities, especially when speed is essential.
For a long time, this data was deemed unusable - consumers don't provide feedback neatly. This is true particularly of gamers, where in-jokes and complex references are very common, but to a lesser extent the same applies to any industry.
Only recently have advancements in AI have made it possible to create a solid model that processes and deciphers this kind of open-ended feedback. We spent a lot of time and compute on this, but here's a quick view over a few of our test cases:
>> TEST CASE 1021 <<
INPUT:
So no factions for part 2 ?
OUTPUT:
I'm disappointed that there won't be any multiplayer mode
in the second game.
>> TEST CASE 2613 <<
INPUT:
At least we got “itchy tasty”
OUTPUT:
I'm glad they included the iconic line "itchy tasty" in the game.
It's a reference to a famous line from the original Resident Evil game.
>> TEST CASE 3161 <<
INPUT:
2030: GTA V Collector's Edition
OUTPUT:
I'm joking that the game will still be being released
in the future, even though it's already been out
for a long time.
>> TEST CASE 3798 <<
INPUT:
Thor “ Are you a calm and reasonable person”
Kratos : “TF YOU JUST SAID TO ME ?? “
OUTPUT:
I think it's funny how Kratos gets so angry
when Thor asks if he's calm and reasonable.
It's like Kratos is like, "What did you just say to me?!"
Yet, even armed with clean data it's not an easy task to turn it into insights. When listening to consumers, you need to not only know what they're talking about, but also what their sentiment is, how important each point of feedback is to their overall engagement with your product and how adapting your product to their feedback will change their perceptions.
So it comes down to thee steps:
- Find a source of authentic consumer feedback
- Run that feedback through a smart analytical framework
- Draw actionable insights to guide your product and marketing strategy.
2. The Framework
The second important step is to know what to listen for. This is where the framework comes in. It's a set of rules that define what to look for in the data and how to interpret it. We devised a set of categories that are applicable to the gaming industry, but the same approach can be used for any industry.
Without going into too much detail, you need to define categories that help you figure out how you're doing (e.g. do your customers think the game is unique) and why (e.g. do they think the game is unique because of the story or the gameplay). Then you track the sentiment or more likely the alignment to the category for each commenter.
This will let you know what your strengths and weaknesses are and what you need to focus on to improve your product. It also lets you compare to your competitors and see how you stack up against them, providing much needed context. A priori, there is no way to tell whether any level of uniqueness is good or bad, but if you can see where other games in your category were, you can tell what to aim for.
You also need to define categories that help you understand the why - what your customers want - for gaming, do they like the graphics? How about boss fights? Or the story? This will let you know what to focus on when developing or communicating your game (or plan your next).
So now you know how you're doing and a little bit of the why. For a real analysis of the why, you need to go deeper, and we provide several (we think) pretty ingenious ways to do that - we analyze topic clusters hierarchically to drill down to reasons, do both extractive and abstractive summarization, and we provide a retrieval-augmented generation powered question answering system to interrogate opinions.
The thing is, it's still not enough. To complete the circle, you need the importance of each category to your customers. This is where the framework really shines. Ideally, you have some outcome data that helps you calibrate what your customers actually value.
Finally, UX is really important - to make so much insight usable, it's vital that you know how to present it. You really need domain experts at this point, who will provide a link from this data to the questions that you need to answer to make good decisions.
3. What we learned
We built TRAILR to do this for the video games industry - within the scope of this project, we built an automated data pipeline that feeds an analytical framework and ultimately delivers performance metrics to the end-user in an interactive dashboard. Within the system, users browse through real-time gamer feedback to upcoming game releases - over 12MM comments across 1K games power the system to date and are run seamlessly through the models.
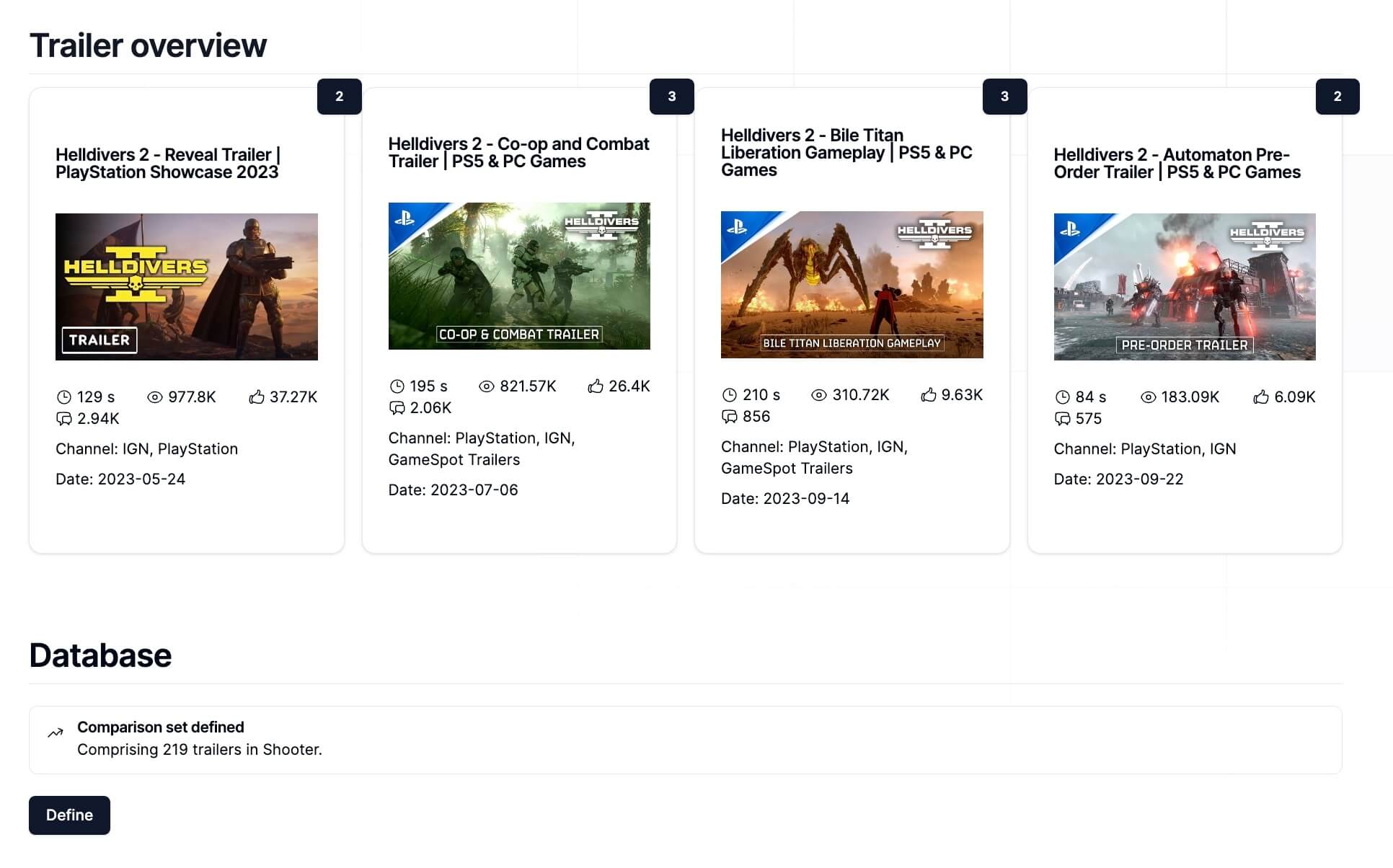
There are a few core learnings that came from developing this system:
- Be meticulous about data quality and the depth of your analytical framework - only measure what you need, when you need, and then use every data point to draw an insight relevant to your business
- Only a machine can remove bias at the scale needed to do real time consumer listening - and do so consistently across time to allow you to track progress
- Know what to listen for - there is too much noise that you need to be able to filter in consumer feedback. Knowing what's noise and what's core feedback is vital.
- Track the feedback of your customers, but also benchmark it vs. the feedback your competitors receive - no metric is useful without an anchor against which to interpret its value
- Context is king - teach your tool to understand and resolve all references and symbols within a comment in order to know what a piece of feedback means. Inject context where needed, but no more than is necessary.
The last two points have been recurring across our projects and warrant individual deep dives - understanding the meaning of a data point and knowing how to draw an insight relevant to your business from it are the two hardest and most necessary aspects of using data to derisk your decisions and processes.
If you're considering consumer listening for your business, don't hesitate to chat with us! We have deep experience implementing this across industries and will help you find the optimal way to implement it in yours.