Case Study - Detect Watermain Leaks from Space
Drinking water loss is one of the most pressing problems of our time, and we lose 35-45% of it globally. We need to act quickly enough to make a difference.
- Client
- Internal
- Year
- Service
- Data Engineering, Artificial Intelligence, R&D
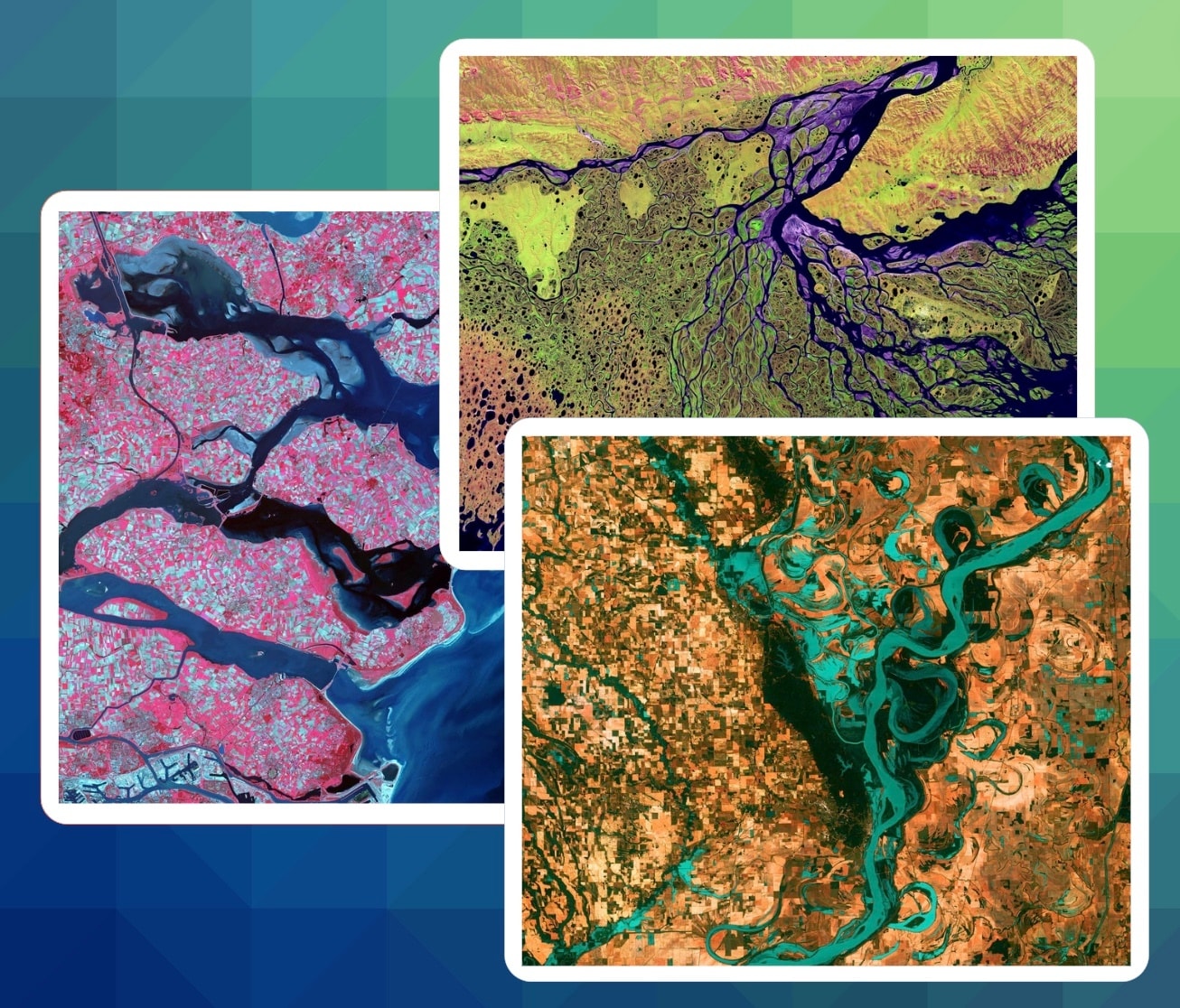
Overview
With the climate emergency, access to clean, drinkable water is becoming a challenge. There is a growing global consensus that water needs to be a lot more carefully managed, reflected in the UN's sustainable development goals and the EU's water framework directive.
We started this work after learning that watermain leaks are a major source of water loss, and that the current methods of detecting leaks are expensive and ineffective. A skilled team with the correct equipment can survey around 10 kilometers per day, which means water utilities can only afford to cover a small fraction of their network each year.
We wanted to build a system that could detect leaks at scale, be deployed globally, and require no expensive maintenance or large investments.
On the data side, we use satellite and remote-sensing data covering SAR, Thermal and Visual in 25+ channels, across time. We can optionally fuse multiple remote sensing instruments, incl. UAV, Aerophoto and pipeline-installed sensor data. Our data augmentation pipeline is fully automated, and continuously evolves its own dimensions (we have over 1k useful dimensions in our models so far). We then use AI / machine learning to detect leaks and optimize the search area for human teams.
We calibrate local and global models using ground truth data from water utilities, and can detect leaks with a high degree of accuracy, even when underground or under foliage, including in hard-to-reach areas. More importantly, we can detect leaks before they become a major problem, and can significantly optimize human survey efforts.
The system is currently in closed beta and we are working on live trials with a number of water utility companies. Our goal is to have as much geographic coverage as possible, so if you (or someone you know) are interested in participating in the beta, please get in touch.
What we did
- Remote Sensing
- Geospatial
- Data Engineering
- Machine Learning / AI
- Infrastructure
- Datapoints per pixel
- 1k
- Water network in EU
- 4.2 M km
- Development time to MVP
- 10 months
- Sens / spec on large leaks
- 100% / 99%